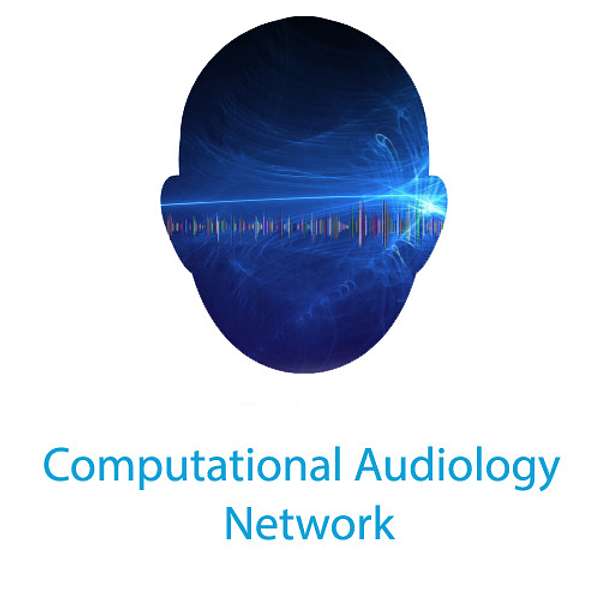
Computational Audiology Network (CAN)
Computational Audiology Network (CAN)
Bayesian Active Learning in Audiology
Here we discuss with Josef Schlittenlacher (ManCAD), Bert de Vries (TUe) and Dennis Barbour (WashU st. Louis) the potential of Bayesian active learning in audiology, in medicine, and beyond.
Quotes from the interview:
Dennis: 'No Bayesianists are born, they are all converted' (origin unknown)
Josef: The audiogram is the ideal testbed for Bayesian active learning.'
Bert's favorite quote: “Everything is the way it is because it got that way” (D'Arcy Wentworth Thompson, 1860--1948)
The later quote reflects on the idea that everything evolved to where it is now. It’s not a quote from the Free Energy Principle but it has everything to do with it. The hearing system evolved to where it is now. To design proper hearing aid algorithms, we should not focus on the best algorithm but rather on an adaptation process that converges to better algorithms than before.
Further reading and exploring:
- https://computationalaudiology.com/bayesian-active-learning-in-audiology/
- https://computationalaudiology.com/for-professionals/
- Audiogram estimation using Bayesian active learning, https://doi.org/10.1121/1.5047436
- Online Machine Learning Audiometry, https://pubmed.ncbi.nlm.nih.gov/30358656/
- Bayesian Pure-Tone Audiometry Through Active Learning Under Informed Priors, https://www.frontiersin.org/articles/10.3389/fdgth.2021.723348/full
- Digital Approaches to Automated and Machine Learning Assessments of Hearing: Scoping Review, https://www.jmir.org/2022/2/e32581
And I think during one of the courses I followed, I met Bert he gave a course about Signal processing in Delft. He was invited lecturer. And ever since we stayed in touch. When I started thinking about computational audiology and using machine learning, Bert already knew the work by Dennis Barbour. By writing this computational audiology perspective paper, I met Dennis and I exchanged nice ideas about the potential of active learning. And Josef, we met each other for the VCCA last year, via Tobias Goehring, I already had seen some of your work when we were writing this computational audiology paper and more recently for scoping review. We have looked into all the digital approaches or automated audiometry that have been published since 2013. And I wondered how many groups would be working on machine learning. Audiometry and yeah, we found the three of you. But we didn't find any other groups. And now this scoping review that's just been accepted and published and I thought it would be a great opportunity to talk with the three of you about what developments you further expect. And one of the reasons for me to write a scope and review was also. Better understand the barriers. Why is it not used in the clinics yet? And how does it compare to other automated audiometry approaches? So that's briefly for me, the motivation to contact you. And I'm really glad that you all replied positively. And so for this interview, I think with the questions and answers, you already provided, we have enough to help to fill a block and to share thoughts of the potential and further development. So having said that maybe good, Josef, if you further introduce yourself and then Dennis and Bert can maybe explain more about their motivation as well. Yeah. So I'm a lecturer now in Manchester, before that I was a postdoc in Cambridge. And before that I did PhD in psychology in Germany and studied electrical engineering. So that's my background and machine learning audiology started in Cambridge on the grant for Bayesian active learning, applied to what Brian Moore and Richard Turner do. Yeah, that's basically my brief background. I think I've met Dennis and Jan-Willem at the VCCA. And Josef, anything for this meeting that you would like to get out of it or your motivation to join this? Yeah, I think it's a fantastic idea that you do that the Jan-Willem because we have our scientific publications, but when you write the blog you might bring it closer to clinicians and even companies so that they finally start implementing it. Yes. And I think that can be really something you can do with this blog, that some of your papers, also the conjoint analysis paper by Dennis, for instance, it's quite dense to, to read. And I think for many clinicians, yeah. If you're busy, you won't be able to read it and think, ah, this is something we need to bring to. Great. Then would you like to further tell us something about your background? Sure. I'm currently a professor of biomedical engineering at Washington university in St. Louis. My educational background is electrical engineering, biomedical engineering, neuroscience, and medicine. I also have an MD and my lab that I set up at WashU was the primate neurophysiology lab. So we were recording neurons in the auditory cortex and trying to understand function complex vocalization processing, and a vocal primate species. And as we started doing a little bit more with humans trying to replicate some of our findings, not oh, we did some electric electrode recordings, but mostly with behavioral data we became interested in perceptual training and trying to induce therapeutic changes in brain function for improving signal detection, specifically speech processing. And as we started thinking about what that, that literature, if you know, that literature is very confusing in the sense of the ability to achieve perceptual training effects that are persistent and transfer in any complex domain. It's very spotty. It depends on the lab and the preparation, and it's not a highly reproducible set of data essentially in the field. And so we started reasoning, how can we optimize the training trials for each person? And that turns out to be a very hard problem, but we stumbled across this idea of active learning for not therapeutic purposes for training, but for testing. The opportunity to speed up testing procedures really seemed to present itself with this set of tools that we were using. And that has taken over what we do because we're not working on training at the moment. We're not even working on monkeys anymore. We're really thinking about and this is why I also appreciate this, the idea of a blog entry that might get things closer to clinicians. We think that now the way I'm contemplating this is I still want to get back to training, but very complex latent constructs, especially that bridge perception and cognition like speech processing. There are many points of failure in noisy speech processing that can happen from the ear to the brain, right? And those are those fall in between perceptual and cognitive phenomena. And I'd like to build models and testing regimes that can bridge those gaps and the amount of data required using classical methods, it's just prohibited for a unifying, that kind of thing. So my goal nowadays is to try to extend the audiogram was just our test case that we. eIt turns out to be quite successful, but it was really just us showing that are proving to ourselves that this approach would work and add value and also kind of giving us some background to be able to do more complex model construction. And so that's my interest in this meeting would be exactly to take those ideas out and promote them as a possible future for behavioral testing in a wide variety of fields. That's cool. And also you mentioned, I think if I would translate it to clinicians is inducing a change in the brain is what we do with cochlear implants when we're starting fitting them. A big problem is that what we do during the first month really changes the brain so that you can not just say, okay, we go back and do it again. There's like a hysteresis loop that you have made changes and people have become accustomed to something. And that's a problem. I think we are not able to tackle yet in the clinic. So Paul Govaerts from Belgium, he did this review about fitting procedures and turns out that every clinic had their own procedure and many of these procedures turned out to be fine because the brain adapts apparently to this. So in terms of trying to relate to what clinicians experience, I think this is an example. And the other thing is that you started with the audiogram and in our scoping review, we found that the mean testing times are around five minutes for a test. So if you're talking about time efficiency, then there's not so much room for improvement. And that could also be a reason that maybe the clinicians are not. Tempted too much to adopt this. But if you show that you can combine it with other tests to speed it up and with complex theory, that might be a stronger case. That might be a more succinct way of summarizing my interest is joining the audiogram to other relevant tests, into a unified or mostly unified testing procedure that integrates the data across all the tests to decide what the optimal sequence of queries is going to be. I guess there's also a bridge to the work by Bert and fitting hearing aids and the complexities they're experienced. I think by clinicians, Yeah, so shall I introduce myself? Yeah. Okay. Yeah. So I'm Bert de Vries. I work as a professor in electrical engineering signal processing specifically in at the technical university in Eindhoven. I still have a small affiliation with GN at the time that we wrote the work on the let's say, active learning for the audiogram. I worked in much larger capacity for GN and then currently. So my interest is in designing, basically automating the design of algorithms, right? The brain is not born with the capacity for speech understanding. We learn to understand speech well, basically through spontaneous interactions with our environment. Then we learn to walk and to recognize objects. There's a beautiful theory by Carl Friston on how the brain computes it's got the free energy principle. It's a very Bayesian probabilistic theory. And my goal at my lab here at the university is to translate those ideas to engineering, to build agents that learn purposeful behavior just naturally through spontaneous interactions with with the environment that could include speech recognition or object recognition, but may also be for robots. The active learning paper. It just seemed since my, our approach in our lab is very Bayesian and it seems this was around 2014, that had a PhD student, Marco Cox, and and in a discussion, we just figured that. Well, this audiogram taking seems like a classification problem. You have a discrimination boundary and everything below is one class. You cannot hear it and above you can hear it. So let's just build a Gaussian Bayesian classifier for this. And and he did, and yeah, it turned out that Dennis had the same idea or at the same time, maybe even a few months earlier. And then so then we we Marco wrote that paper and put it on arXiv and we found out that Dennis had basically written about the same paper and yeah, so that's the story. And then it took a long time. I think recently Marco made some improvements to his design with a new Prior and a mixture of Gaussian processes. And that's our paper, 2021. Okay. I missed that. Didn't read the update yet. I was also surprised about you bringing up the Free energy principle by Friston and yeah, for what I heard so far about, try to understand about it was it's quite complex in also using it for actual predictions, but that's, that's some of the critiques, I think, on this model, Okay, so the idea here is that what Carl Friston says is the brain follows just the laws of physics and the laws of physics. There's sort of an umbrella framework for describing the laws of physics. That's called the principle of least action. You can write down a function or functional. And if you minimize the functional, you can derive mechanics, classical mechanics. Electrodynamics basically all the branches of physics. And you can also do this in sort of an information. You can write the information, theoretic formulation of this, and then it turns into what we call the machine learning variational Bayes. And he claims that's all that's going on in the brain, just following the laws of physics. It just turns out that if you write that down in an information theoretical way, then you're doing also Bayesian reasoning. So following the principle of least action in the brain leads to Bayesian reasoning, which is machine learning. And so you can use it then for yeah, for information processing, for designing algorithms for all kinds of stuff, for learning how to walk and learning how to hear. And the, what we do in my lab is not to verify that claim. But use it as an inspiration for engineering, have an engineering lab where the design of hearing aid algorithms and fitting of hearing aid outcomes is an interesting application area. But the principle is broad enough to include lots of other applications you could think of self-driving cars or robots subtler, and to walk in the, the key observation is that since this is like an umbrella kind of theory it's basically a one solution method to all the problems, right in engineering, when you started a problem, and then you go to the literature, you find 15 solutions and you modify one, and now you have 16 solutions and the brain turns that around, but I cannot afford for every problem to come up with a new solution method. There's just one solution method. Free Energy minimization or following principle of least action, one solution method that learns both the problem and its solution simultaneously. And it also scores how well the problem is represented and how well the solution solves the problem. So there is one cost function for all problems and that's, that makes it really nice also for engineering, because I can apply it anywhere including to, to audiology if you, if you want, but also to other areas Another way to say this is that you apply probability theory then to problem, or is that an oversimplification? Well, this is a simplification, but it's also very accurate. And that's also, I mean, I think in, in, in essence, The three approaches by Dennis Josef and, and Marco, because it's mostly Marco's work. Marco Cox's work very similar it's so it's Bayesian classifier for an interesting problem, you know finding an audiogram. But that framework of active learning could really, is really broad, can be applied to much broader sets of problems than just to to taking an audiogram. Yeah. I think also this was one of the questions that was, yeah. Josef, I think you had the question at a similar applications outside audiology, and you also started with us, are we constrained only to the audiogram in this discussion? So I guess this question already is coming up here naturally, and I guess the three of you all have your own. Thoughts or directions on where you are heading towards. Is it an idea that you briefly mentioned this future directions you're aiming for or applications you think are interesting? Josef would, you start? Yes I can start again. Well yeah, the audiogram is the sort of the ideal test bed because it's simple. It has those two dimensions. One of them is distance based. So it could be even more than one dimension, just something that gives you distance that's frequency and the other dimension level in the classification problem is that monotonic dimension. So by having these two dimensions, it's the ideal test. And as you said we can put some lots of effort into it then decrease testing time from five to three minutes or even two minutes, which is from a scientific point of view, exciting from a practical point of view. It's not too much, so it's an ideal test. But we really want to use it for further tests. And within audiology, there are lots of further tests that can be done. Like Dennis said speech tests With speech you have the problem that you have not two variables, but many, and you have to identify which one do you want to learn. And so, and we have done a similar thing with the notched noise tests, notched noise test. You have about eight variables, signal frequency, you masker frequencies level. And so on. And eight, eight variables are too much the curse of dimensionality just hits. And so you have to tailor the problem to reduce your variables. What we did, we reduced it to three variables, but one of them was signal frequency, which wasn't done it notched noise test before you typically test one auditory filter at one frequency. But the huge advantage of the Bayesian active learning is like in our audiogram approaches. When you have a continuous frequency, then suddenly you get the auditory filters across the whole hearing range. And then suddenly you have a huge advantage because testing several auditory filters and four or five frequencies takes two hours or longer. And this with active learning, you can do it in half an hour. And that's a real difference for the clinic. You can put the patient in the booth for half an hour, but not for two or three or four hours. And the audiologist doesn't need to be present during the half hour. Those tests are automatic. They can correct for errors because everything's probabilistic. Figure out that one answer is so unlikely that it was just a wrong button press. So that's the beauty of these tests. Yeah, we have worked for a few further tests, like auditory filters, dead regions dead region was without the Gaussian processes, but also Bayesian equal loudness contours. There are probably many more to do. And I think the three groups of us will work in slightly different directions and provide many more tests. So that's great for audiology, but we should keep in mind that there is broader field of application in the whole field of healthcare, where you ask patients questions where you do more than one test or can do more than one test. Then you want these Bayesian approaches. For example, when you measure your blood values, then you need to take a needle. So that makes it less good than as a test bed. And then you can choose which vallues you want to analyze, and that costs money. So if you have a Bayesian approach for that tells you which values are interesting to analyze, the doctor's opinions, that that sort of thing is where we as audiology could be the pioneers, because we have such an easy test bed and other fields of medicine could identify where they can use our approaches and integrate it into their practice. Yeah, that's a really cool broader perspective. And I think to have to also return to this in this discussion also since this is what you mentioned with, by pioneering really a paradigm change in medicine if you would do this. And so I would like to discuss this further, but I think Bert and Dennis, you also have ideas for the applications. So Dennis, what further applications are you considering? Yeah, I'll say I just agree with that assessment. 100%. I keep saying this in talks, like all of those points that Josef just made and I think they go over most people's heads. I really believe audiology can be an example for the rep because our problems are tractable. I won't call them easy. But they're attractable for these approaches and the same kinds of approaches could be postulated in other fields. It's just harder to think how to state the problem in a way that's actually a solvable in the same way. So we're starting that direction for, I mentioned earlier, my interest in bridging perceptual and cognitive constructs. So we're starting now building cognitive models in the same way. And it is considerably harder than psychophysics. And it's because the the feature space comes for free in psychophysics, but in cognitive spaces. There's no even general agreement about what the feature space, what is memory can only be operationally defined and everyone has their own operational definition. That's to give you an example. So we're generalizing the the principles of the model construction, the active learning, and then the population level analysis. I'll talk about instruments to these, the latent variables. We're trying to generalize what we're doing to active, latent, variable modeling as the way to say it, I would say, and how we form these latent variable models. It's not going to be as simple as pulling off the shelf kernels, like we've used so far for this this probabilistic classifier. So we're trying to figure. Ways that we might do that both empirically from data and then from theoretical constraints that we can impose on the problem from other knowledge and we're making progress, but it it is so much easier to operate in perceptual space. We're still keeping projects alive there because we can make progress. And I've the machine learning audiogram that I've beaten into the ground is because I partly, it is such a great model system for it's like the audiogram I think of is literally a model system, right? It's a use case that has a gold standard. It's got, it's the simplest complex kind of psychometric functioning you can postulate. And it just makes a great test bed for, you know, can we speed up things? A factor of two or a factor of four. And if they don't work for the audiogram, I wouldn't spend all this effort on trying to build these more complex, latent variable models. So I think the next step for me is where I think we're going to get the biggest payoff for these methods is in procedures or diagnostic paths, trajectories for disorders that are highly variable within the population. So when there's great population heterogeneity, you can't just average across big cohort. You can't just take a little data from a lot of people and then plug new patients into this population somehow and understand the best way going forward. So we in audiology also realize that because every fitting procedure is at least a cochlear implant level. And I would already do, even from the hearing aid level, everything is individualized in a sense, you can't just blindly pull plans, rehab plans off the shelf and apply to everyone. So we already have this mentality that things need to be individualized, and that doesn't really exist throughout the bulk of medicine. Even though the concept of precision medicine is all about adapting your therapies to the individual. It's just not being thought about in the same way as we in rehabilitation, we think about it. So my goal is to expand these tools into a space where we can conceptualize more complex, latent constructs in brain and behavior. So I'm not going to leave brain and behavior. Because I think there's plenty of space, plenty of work to be done there, but I want to use all of these as templates for the rest of medicine. To say, oh, these active learning procedures are, are really useful when you have highly variable manifestations of disease. And we can reduce the amount of data that we need to collect from each person to make a diagnosis for them, and then ultimately decide on the optimal treatment. And it could vary. You might, these methods might ultimately lead to a rational selection of completely individualized treatments for each individual. And without having, you know the way the clinical trials work is you give a cohort, an intervention and if it works, then the range at which that intervention is deemed to be relevant is who was in that cohort. So we're trying to break out of this population or cohort level rules of inference and bring in the formal ability to infer across a variation in populations and still pick the best choice for them. And I think these Bayesian methods are ideal for that. Well, I think Josef just explained a paradigm change that we need, but you also now run into the same problem. I would say that for instance, these cohort studies also to get FDA approved for a CE marking, you need to test something on a group and as clinician I should say that often we just have a single solution. For hearing aids there's a prescription rule and you more or less, it's a one size fits all you give to almost everybody. And sometimes it's some tweaking, but then you run into a really gray zone that you don't really know what you're doing, and it's based on previous experience. But I think if you asked clinicians their fair assessment of how certain they are, what they're doing. It's probably either trial error or something that they've done before and it worked on that particular patient. So they just try it again. And that's also sometimes I think what for the clinicians, is their reason to be like, this is really where they excel like, oh, I know this experience from the cohort. This person is really different and together the patient and I will look for a decent solution. Or if you give up after a couple of trials, you'd say, okay, now we start to counsel on how to cope with this limitation that we cannot solve. And I think what you propose would help the clinician in this search. But it's also a leap of faith in the sense that would, I as clinician also be able to understand the procedures that I'm following then or it's kind of black box that's providing me advice and would a clinician still be needed or is it better that the algorithm directly interacts with the patients? For instance? My brief answer is I absolutely believe clinicians always needed. Clinician and patient and maybe supporting family need to be involved in the decisions. The Bayesian methods can provide guidance and suggestions, but they don't provide value. Right. They can't, I mean, you can define a cost function, but that's outside the scope of the algorithm, right. That has to be defined by the people involved. And so these are clinical decision support tools. And that's the right, that's the terminology within AI in medicine. And that's the right terminology here. They're here to very complex scenarios where the human brain doesn't log conditional probabilities, well, which we don't do very well. You, you rely on the algorithm to compute those things and then evaluate at the outcomes level, essentially. So then for working out this interview, it's important that we stress that point of why clinicians are needed, but also what change in mindset or approach for clinicians is needed and how to get them curious to do this. And what I maybe can add here is that for the website, we see a lot of people from all over the globe visiting the website also from many countries in Africa or Asia. And I think that there is curiosity, but also fear in the sense of so for instance an audiology trainer who was a little bit depressed that she told me that, or she shared on LinkedIn that many of the students were uncertain, what kind of job they would get. And she actually made a call. Could you please share positive experiences of how your job can develop and the opportunity? So it's more or less on my to-do list to say there's a lot of things that you can explore to improve your clinical care. And we just wrote this Wikipedia article about computational audiology. And what I like about it is that I learned that my one sentence summary would be translating models into clinical care. And I think also with Bert de Vries, two years ago, already this discussion. He said that it's a model based approach, but this translating into clinical care. Yeah. It's of course really important that clinicians there are involved in and see the potential. You know, Jan-Willem I have a suggestion actually, because I have had, I believe five audiology students come through my lab and publish papers with me including that very first paper. So maybe, and I have found that the younger students are very interested in this technology and see older practitioners that are the most skeptical. So it might be interesting to interview students who have worked with these automated and or machine learning based methods and get some of those interviews up on the website. I think that could be interesting. Yeah, that's a good idea. Then let's continue with this question to you Bert especially since I think we are making this application space bigger and bigger, and probably what you mentioned already, what your lab is doing. It's the biggest space more or less. So how would you define the applications and and how to use the scope of maybe medicine then, if you say that audiology is maybe some kind of example case, but the real benefit will be when you do this in medicine at large or society at large. I mean, I first I completely side with Josef and Dennis have said, right. I'm not an audiologist. We are all three by training originally electrical engineers. So you need to keep that also in mind, right? So we have a very computational view on this whole field, and that may not be the best for, I mean, Dennis is also a physician, so that's, he's maybe different. But so in my view, I mean, just, I have a few comments also about what I just heard. It's important to remember that if the audiogram just tries to estimate the hearing threshold, but the hearing threshold is not something physical in your brain, it's a variable in a model. It's just a variable in a model that we write down. Right. And with that model, we can say the model that can predict if we give a stimulus to a user really answer or not, and all the Bayesian method does is, well, it provides a framework for estimating that variable, but the Bayesian framework is broader, right? It can estimate any kind of variable. So my interest, the next step would be, oh, let's estimate more parameters in the hearing aid algorithm. In my lab, we are really interested in using the Bayesian approach. So not just estimate the parameters fitting, but just estimate or derive the whole algorithm. Let's just do I have the whole hearing aid algorithm. So that's a long-term ambition that will take years and we'll see how far we get. But in principle, there's nothing that would stop us from doing that. Having said that there is nothing that we do here. We never see a patient, right? It's sort of an isolated exercise here that we hope at some point clinicians can use and take to their patients. Right? I mean, none of my students will ever see a hearing aid patient. We have no idea. About how to deal with hearing aid patient. We do technical work. It's very interesting. And I think there is a chance that with the work that we do, that I should just move about and you interact with an agent which I like what I'm hearing. I don't like what I'm hearing that over time, we can design a hearing aid algorithm, how that is used by a clinician who is talking to his patient is a completely different profession. So my advice would be for audiologists and clinicians, try to stay interested in what's happens on the technical side. I mean, you don't just to know the details about Bayesian inference, but Bayesian inference is an important growing field. It's really interesting to learn something about it. I mean, there's a reason why we all three are so enthusiastic about it, and it's very important also for I think for audiology. And just reinvent yourself. And I would say the same thing for signal processing engineers in the hearing industry, because if this works, if we build agents that design algorithms, then what do we do with the signal processing engineers? So it's not a problem. It's a problem for all of us. And also for myself. So we all have this problem. I think of a bit of anxiety about the future. And probably the soup will not be eaten as hot as it's served. So that's calmed down. It reminds me of one of my favorite quotes actually never have no fear.
It's from the movie:'The Croods'. It is very fun to, to watch about a family in the stone age living, and they are afraid for anything. But what you're saying now is I think maybe people reading about your work will think, ah, okay. I need to find a faster way to get to the thresholds. But the reason in the first time we were measuring thresholds is that it's too complex to measure responses of people, to all sounds cause that I think you would want to do optimize how people hear any sound, but instead we were able to measure thresholds and then use the half gain rule more or less how much gain ,amplification, of sound we could provide our patients. And I think we have even forgotten about the ideal of making any sound audible and another thing maybe that's you are now, touching upon. How to get to ecologically valid assessments, because what we do is measuring in a sound booth, a really artificial sound, only pure tones or maybe warbles. While, you would like to know how people are actually hearing in their daily lives and how to optimize it in that situation. So that's of course also really close to the hearing aid fittings, I think in optimized situations. Yeah. No, I think eventually, eventually I think most of hearing is fitting should take place in the field where the problem's happen, right? Yeah. I mean, you don't want to fit anything until you have a problem and then you solve the problem and you move on, then you solve the next problem and you move on. And this is sort of how we Bumble through life. And I think it's also how we should teach our hearing aids, how to behave. So, and in principle, you don't need a hearing threshold. Let's say you don't need in principle pure tone audiometry to estimate the hearing threshold. You can, if it's a variable in your model, and then it's just a latent variable and Bayesian methods. If you get responses from patients to other stimuli, you may also be able to estimate that hearing threshold, and maybe you will find out that in order to listen well, to sounds, we don't even need a very accurate hearing threshold. Other parameters may be more important, right? So the hard focus on getting the most accurate hearing thresholds is something. I think that over time be less important. Yeah, but I'm not sure if the others agree with that, but that's how I feel about that. Can I say quickly, I agree. And I have focused a lot on these hearing thresholds the autogram, but I view that as a proving grounds, right. For being able to incorporate more interesting latent variables, I would love to eliminate the sound booth entirely because of the ecological validity question. Right. That just got issues there. I agree. I agree. And I think maybe one of you also wrote it in the answers already. I guess we clinicians also have the term the hidden hearing loss, which shows that we cannot measure this, but once, we would use active learning. I guess we will be better able to pinpoint these cases of hidden hearing loss, where we don't have the sensitivity to detect hearing difficulties and that we have this difference between what the patient reports in problems and what we measure in our sound booths. I'll think in terms of time one of the challenges was that you could also of course ask each other questions. Shall we make a round of questions to one another? Dennis, do you have a question for Josef, for instance? Well my, my original questions were origin story questions. Like how everyone in this group kind of got into this mode of thinking, but what I've already taken away is that I think from slightly different perspectives, but maybe not so different since I didn't realize we were all trained in electrical engineering and signal processing. I mean, I considered myself a signal processing engineer, I guess, for, it's why I got into auditory work at the very beginning and then ultimately into the neurobiology of hearing later. But I feel like I've, I know that better now. So it's, there's some uniquenesses, but I think we're integrating other ideas out in other fields and taking them to this space and that I guess I just had a comment that was interesting to hear. And I would have asked that question if I didn't feel like I got a pretty good sense of it already. But maybe another question then, or Josef, do you have a question you want to ask Bert or a Dennis? Showing all their future plans and use cases in great detail. And I just agree with all the motivations then. As a comment, I mean, our approaches are a bit different, especially with the future plans. I mean, Bert approach is big data. And with that free energy and variational Bayes that's a very interesting area because he can handle many more responses, much more data with those approaches. And I think that variational Bayes has not been done too much so far in our applied Bayesian active learning field. So I think that's a very interesting work that he's doing. Oh yeah. And then so say that was a surprise that there was a lot of alignment between the three of you. Well, of course for the approaches that's not a surprise, but also in, and maybe I should have invited another person or clinicians to get this better, this contrast on what's used in practice and what new tools are developed. So your ideas of maybe also doing some interviews with clinicians, I'll think about it. And what I also wanted to share is that in November there was a reading group about computational audiology, which was initiated by an lecturer in audiology from Texas. So she was interested in machine learning in her field. And so she contacted me in, I think, October and we put it on the website and then. Eight people responded and followed the same course on Coursera about artificial intelligence. And the eight of them had discussions on Sunday every morning Sunday morning in that month. And that it's really nice discussions. We, they were done in slack and we've got people are just typing in the responses and thoughts about these lessons. And she had prepared always two or three questions for the week based on the course that with the whole group was seen more or less in the same timeframe. So there's a, I could start with asking them their thoughts of how they tend to use these ideas into either a clinical training or in a clinical work. And it shows that it's maybe still a tiny group, but there is people really curious about these new methods. Other things you want to share? Or otherwise we can sign off nice in time. I do have a question maybe for everyone. It's interesting. I think that the three of us have converged in this particular space and I would say hearing science have you had any, have you seen similar work going on in other fields that is parallel and I can say in vision, we just got a grant to do the same thing. A vision test they're Bayesian methods that have been used in other psychophysical domains, but this exact kind of approach that we're taking, I just haven't seen elsewhere. I'm wondering if you guys have seen that kind of thing? No, not for a very long time. And there's that paper in 1999, Kontsevich and Tyler who did that vision thing, who basically said that we are doing that way, which we are doing now, but with the computation of the 1990, so much simpler. But yeah, after that I haven't seen too much in that field. Out of personal interest, I tried to reach out to rare diseases and auto immune diseases, but so far without success. So I tend to send pitches at my university and other UK universities. I think in most fields, there is a sub community of Bayesian scientists or engineers. We try to approach the classical problems in their field from a Bayesian few point. Like for me, I got interested in a Bayesian approach around early two thousands, like 2000, a one or two. I'm not so sure by reading articles from these theoretical physicists who were applying it in cosmology because in astrophysics experiments are extremely expensive there. So they have to do active learning. They have to make sure that their experiments are informative. And and then I thought, well, we work with people, you know, our experiments have to also be informative. So that made sense. And since then I started working on Bayesian methods and after the initial, maybe it took like two years for me to realize that, wow, this approach covers basically the whole scientific endeavor, right. Bayesian approach is basically a description of science. It should be part of every field. And in every field, there's a sub group of people working on this also now in this sort of if we're hearing aids, but I think in almost every community and some communities, a little bigger group in other communities are very small. But I would encourage people to study it. And the papers that we wrote, I mean, it doesn't matter whether you read, I think Josef's paper or Marco's paper or a Dennis' paper, it's a good test case. It's a very clean problem. If you studied a little bit of Bayesian material and you can read, then you can actually say, okay, can I read the paper Josef wrote or Dennis wrote? It's a very nice cause if you can, and you can actually understand that paper, you will start to see, oh, but now I can apply this everywhere. These Gaussian processes they are Actually, I think it may be, it could be come from the group that where Josef used to work, Richard Turner. I mean, he applies them everywhere. I think that you even put them on the web and you can pay money and they will optimize your optimization problem, solve your optimization problems. So they are applied. Almost everywhere now. Yeah, but it's still in every field, a very small subgroup of people. That's a good example from Neil and Cambridge, who had that paper in 2011 about Gaussian processes and active learning which interestingly wasn't published but has several hundred citations. I think the machine learning world was skeptical at the time about that. If, if you read it with your audiological view, then the whole paper seems like, okay, you can use it for the audiogram, but then they needed a few more years until Dennis was really the first to publish that and say, yeah, this is an audiogram. This has an application. This is not just machine learning toys stuff in some fancy mathematical words. So a huge credit to Dennis and well Bert at the same time. Yeah, just going back to Bert's statement. My favorite quote in this space is 'no Bayesians are born, they're all converted'. So so it's like most of the people who are real Bayesianists that I have worked with have some kind of epiphany story. They they've been scientists and they stumble across this literature and realize, oh, this describes exactly how I think about these things and no one ever taught it to me. So I just reminded me of that. And, and I, I do agree there, there are Bayesianists operating in these different spaces, but the combination of Bayesianism and the exact models that we're using and the treatment of these psychophysical tasks as classification, problems to solve and the types of models, that kind of conglomeration together, which is where the power, the full power of these techniques emerge. It's still, I think we're leaders, that's my conclusion at this stage is our field is leading the charge because I'm not seeing it at least at this degree in other spaces. Wow. I really liked this. So what I want to ask you, maybe it's good. If the three of you share your favorite quotes that we will put this in the blog. Also what I thought I put it in the chat these resources that since we have been discussing a little bit using this in medicine at large, it's important that people start to get an intuition and use cases and can play around with it. So on this website with the resources so far we've collected. Tools models that can be used for remote audiology or for research purposes. So if you have something that you are free to share yeah, please consider it. There's a lot of different ways we could share it on this website. Thanks. A lot Jan-Willem. It was really a great initiative. I really enjoyed it. And thank you also at Dennis and Josef. It was really good to meet you. This was fun. I agree. Thanks it was really great. Thank you for listening to the first episode of the Computational Audiology Network Podcast
Guests:Dennis Barbour, Josef Schlittenlacher and Bert de Vries
Podcast soundbite design:Steve Taddei & Jan-Willem Wasmann
With contributions and help from:Marc van Wanrooij, Bas van Dijk, Enrico Migliorini, De Wet Swanepoel, Alan Archer-Boyd, Dennis Barbour and Elle O’Brien. Podcast production & host Jan-Willem Wasmann